18. mai 2016
We Need a Data Revolution
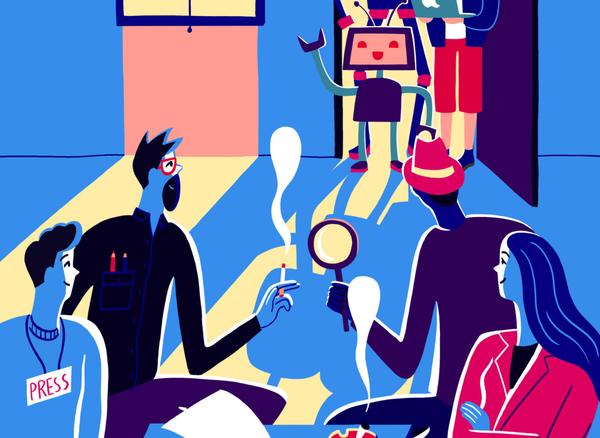
Forget hits—engagement is what will save journalism in the digital era. So why can’t we measure that instead? Over the […]
Forget hits—engagement is what will save journalism in the digital era. So why can’t we measure that instead?
Over the past few decades, media companies have plunged into the digital world with varying degrees of success. While some have flourished, many others are still struggling to discover a business model that works—and nearly all would acknowledge that their profitability is not as high as it was in the print era. Meanwhile, many of the new disruptive forces in digital have embraced social media to drive huge volumes of pageviews, but these new models don’t leave much space for serious journalism that requires significant time and money to create.
Fundamentally, I believe that these problems all stem from underinvestment in data and a lack of deep understanding of media companies’ users. Having spent a number of years working in social media, I can attest that social media companies have a huge advantage over traditional media in their understanding of their users and their ability to leverage that understanding to create a highly engaging product. In order to compete for users’ attention in the digital era, we must match social media’s data sophistication, but in a way that plays to traditional media’s fundamental strengths: original journalism and independent editorial judgement. Schibsted Media Group has a great head start here, and I couldn’t be more excited to help fully realize this revolution in data. But the truth is, in many media companies, this change is long overdue. If the media industry is to thrive in the years ahead, we must join forces in data technology to revolutionize the way the media industry uses data.
Measuring the Right Things
Driving a data revolution starts with measuring the right things. Many companies, naturally, tend to focus on metrics that are directly linked to monetization. Often in digital publishing, revenue is tied to ad impressions, which are in turn a function of pageviews. As a result, journalists are accountable for driving ever-higher pageviews for their articles. Under pressure to chase pageviews, many digital media companies have resorted to creating content that is shallow and quick to produce, enticing users into clicking with misleading or exaggerated headlines. These techniques do drive higher pageviews—for a time, at least. But the user experience is terrible, the pieces are unsatisfying and far too often fail to deliver on the headline’s promise—so rather than building an ongoing loyal audience, these practices inevitably create the need to get more and more new users for each piece. Does anyone believe this is a sustainable business model? What happens to the democratic ideal of an informed, empowered citizenry when this race to the bottom plays out?
Time-on-Site
This trend in digital publishing towards shallow, quick-hit pieces is a direct result of the focus on pageviews. But the good news is that there is a better way. The focus of digital publishing should be on building a large loyal readership—people who make reading digital media a daily habit. And if you want loyal, engaged readers, you need to measure them and understand them. Rather than pageviews, the focus should be on deeper metrics like time spent on site and number of days visited in the last week. These stats give a deeper measurement of user engagement and habits. It’s true that focusing on these metrics as a publisher may cause a short-term decrease in page views, and thereby also hurt ad revenue. But in the long run, this focus on building an engaged, loyal user base will result in more sustainable pageviews and better opportunities for monetization.
The Influence of the Right Metrics
Once the right metrics are being tracked, every aspect of digital products should be oriented towards optimising these metrics, thereby building an ever-larger loyal and engaged user base. There are two ways the right metrics can have a positive impact on digital media products: First, they can guide decision making in product design and editorial judgement. Second, they can guide algorithmic control of specific aspects of the product. Let’s dive into the specifics of each.
Product Design and Editorial Judgement
A well-run data analytics function will produce a steady stream of useful insights about the product’s users. With the right metrics in place, analysts can focus their attention on understanding what types of content and media products create loyal, engaged users. The analysts can then break the user base down into segments representing different kinds of users who like different kinds of products and content. They will track cohorts from each of these segments over time to see what kind of product flows and content result in higher engagement and which result in lower engagement. They will run experiments to verify their findings.
But there’s a catch: This work will be in vain if the product owners, editors, and journalists are not actively collaborating with the data analysts and using their findings to drive higher engagement levels. Journalists can learn which of their pieces contributed to higher engagement, and, in collaboration with the data analysts, learn why their pieces had the effect they did. Journalists can then use this knowledge to create the kinds of content that will appeal to a large number of people and keep their readers coming back for more. Editors can learn how combinations of various kinds of content can work together to drive higher engagement across whole segments of users, and ultimately across the entire user base. Empowered with this knowledge, editors can then plan and assign a mix of stories to give their publication the best chance of growing an engaged user base. Product managers can learn what kinds of product designs and flows will enable users to find the right content easily and quickly. All of these people should be in constant communication with data analysts to continually refine their understanding and experiment with new approaches.
Algorithmic content sorting
Different segments of users are interested in different types of content. Of course, in reality each individual has unique tastes and expectations from media that are slightly different from anyone else. In describing how data analysts can collaborate with different media functions above, I emphasized addressing segments of users because segments allow us to think about the varying needs of different types of readers.
To truly give every user the best experience, we must go beyond segments to individuals. This can’t be done practically by humans alone because there are so many more users than analysts, editors, journalists, and product managers—which is where algorithmic content sorting comes in. Algorithmic sorting can ensure that each user sees exactly the content most likely to engage them every time they visit the site or app. There is sometimes trepidation regarding using algorithmic methods in media. Don’t we risk removing human editorial judgement from the process, which gives each publication its own distinct voice? On the contrary, to be truly effective, I believe algorithms must, like any useful tool, be handled by human editors. Editors, working in collaboration with data analysts, have expertise in the mix of pieces that best reflect both their publication’s mission and the selection of content that will build a large engaged audience. Some content, like breaking news, can be written quickly and cheaply. Others, like longform investigative pieces, take a great deal of time and money. Getting this balance right, in essence constructing the right palette of content available to serve in real time, is a human function. Selecting from this palette to meet each individual’s preferences is an algorithmic function. Optimizing the way that these two work together is how I believe digital media can truly thrive.
Monetization in the Digital Age
Of course, for digital media to thrive, we must also address monetization. For digital media, that most often means advertising, but let’s not forget that building a large, engaged audience of users who visit again and again opens up business models beyond advertising. Notable examples are meters, paywall subscriptions, and micro-payments. All of these models require a loyal, engaged user base to really work. That being said, most digital media is monetized by advertisements so it is worth spending some time addressing ads.
Let’s assume that we have a digital media product that operates as I described above; product managers, editors, and journalists work collaboratively with data analytics and algorithmic content sorting to optimize the product for building a large engaged audience. The currency of such a product is attention. Everything in the product is finely tuned to direct the user’s attention to the content that will engage them most. Now, imagine we put some display ads on the page. What happens? Some users will see an ad that catches their eye and click on it, taking them off the site. We have essentially directed the user’s attention away from our finely-tuned engaging content in exchange for money. And if the ads are not as finely-tuned as our original content is, then we have also decreased the overall engagement of our users in exchange for money. There is nothing inherently wrong with this model, so long as we acknowledge the trade-off and work to minimize the decrease in user engagement. And to minimize that decrease in engagement, we must treat advertisements with the same attention we give to our own content.
Seen from this perspective, the solution is clear: Media companies do not produce advertisements, but they can ensure that ads are high-quality and personalized to the user. To do this, they must leverage the same user insights and algorithmic sorting to ensure the best-available advertisement is matched to each user’s personal interests and preferences. The vast majority of digital media companies outsource ad targeting to 3rd parties, but this introduces new inefficiencies. If a media company invests in data analytics and algorithms as I suggest above, then it makes sense to use these same capabilities to minimize the engagement cost of making money through advertising.
A Global Powerhouse
All of this is naturally much easier said than done. Many digital media companies are driven by financial considerations and simply do not have the significant capital and development time required to build data analytics and personalization capabilities. It is not realistic, or even desirable, for every media company to build the same data functions. In fact, these capabilities only need to be built once if media companies join forces to collaborate on a single publishing and advertising platform.
In this model, the participating media companies would provide the content, the user data needed to power insights, and the ad inventory. The platform would provide the right metrics, the personalization algorithms, and the ad targeting. Each media company would still run their own destination site and app, but the sorting of the content and ads displayed would be determined by the platform. In fact, content from one company could even be shared with other companies through the platform to enable a super-personalization that drives engagement higher than would be possible with a single publication on its own (and with revenue-sharing arrangements to compensate the content creators). In the end, the platform would take a cut of ad revenue, but this would be well worth the higher user engagement and hence higher revenue that the platform enables.
Such a platform would have several advantages over ad-centric platforms like Google’s and content platforms like Facebook’s Instant Articles. Unlike with a pure ad platform, media companies would get the benefit of analytics and personalization services that build a loyal audience. And unlike with Instant Articles, media companies would have full product control that comes with a destination site and fuller flexibility in monetization. Perhaps most significantly, a media-built platform would have publishing as an inherent part of its DNA, and would have a sensitivity to issues like freedom of the press and censorship that are sometimes lacking in the tech industry.
This kind of media platform has many advantages. It enables the kinds of data technology and practices that build a large, engaged user base without the need for every company to build these capabilities themselves, it provides a viable business model for media companies in which they can still focus on their core mission, and it enables editors and journalists to join forces with data analysts to understand their readers to a greater depth than ever before. Significantly, such a platform has a chance to have sufficient scale to be a global powerhouse in digital advertising. As much as the platform would be a boon to media companies around the world, it has the potential to be a huge success for the media company that can build it.
Schibsted Media Group is well on its way in revolutionizing data usage to drive user engagement. But we need all media companies to join us in building these capabilities to secure a bright future for independent journalism.