18. mai 2016
The Importance of Thinking About Cross-Platform Data
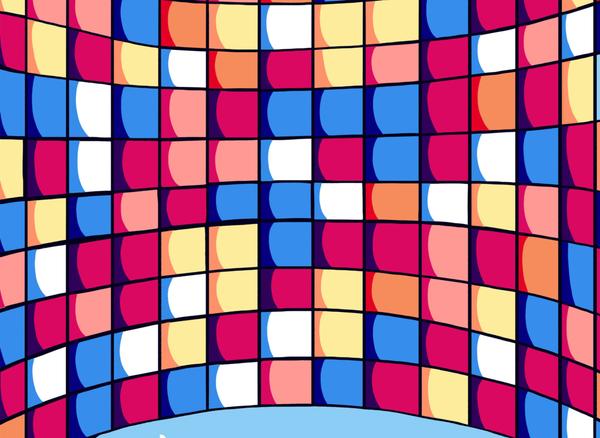
How BuzzFeed approaches data today, and why it’s always changing As publisher at BuzzFeed, I get one question more than […]
How BuzzFeed approaches data today, and why it’s always changing
As publisher at BuzzFeed, I get one question more than any other: What’s the one metric you value most? I’ll let you in on a secret: there is none.
Jonah Peretti, our CEO and Founder, hired BuzzFeed’s first data scientist in 2010 to predict when and how articles would go viral on the Internet. It was, and is, a challenging problem. We are still thinking about this same question today, but a lot has changed in six years. BuzzFeed then was focused on entertainment and viral media. Today BuzzFeed teams in 11 countries publish content from breaking news to scripted shows on over 30 platforms in seven languages. For a publisher charged with collecting and understanding all the accompanying data, the task has never been more complicated or prone to error — or more exciting. Even two years ago, when we all lived in a simpler media landscape, we believed there was no “one metric to rule them all.” Today that is even more true.
BuzzFeed started talking about pursuing a distributed strategy in January 2015. Jonah Peretti, our CEO and Founder, said it best here, but in layman’s terms, instead of focusing primarily on our website and apps, and using social networks as a way to send traffic to them, we began to aggressively publish our content directly to platforms like YouTube, Facebook, and Snapchat. This meant that our daily, weekly, and monthly traffic reports tracking Unique Visitors (UVs) to our website and page views were suddenly outdated. Platforms like YouTube and Facebook don’t regularly provide UVs to publishers, so we needed a new set of data to measure the overall reach and impact of the company.
Internal Unique Visitors numbers (using Adobe or Google Analytics) only measure unique browsers of our website, mobile apps, and Facebook Instant Articles. In the case of analytics firm comScore, UVs measure our U.S. web, app, and Instant Article audience plus desktop YouTube viewers. ComScore UVs as they are reported today do not include people who:
- watch our YouTube videos on mobile (more than half of our YouTube views are on mobile)
- watch our videos and comics on Facebook, Snapchat Discover, Instagram, Yahoo, Tumblr, Vine
- use our website or mobile app outside the U.S.
For a long time, the digital media world was obsessed with driving traffic to their own websites, but in today’s cross-platform world, that metric just doesn’t encompass all of the content we create and produce at BuzzFeed. In fact, We estimate that our current comScore metric of about 80 million UVs represents less than one-fifth of our actual global reach, based on ad hoc data provided by our partners. Less than one-fifth. UVs were useful for a long time. And for some publishers, they still are. But it’s time to stop talking about UVs as a way to measure online audiences. Future media companies who publish on many platforms (like BuzzFeed does) will need to look beyond a simple, one-size-fits-all approach to data, and get comfortable with the more difficult and chaotic world of data in a platform environment.
In order to do this at BuzzFeed, we’ve had to scale up our data science team, currently a team of 10 data scientists (and growing!) inside our tech group of 180 engineers, product owners, and designers. The scale of our data has increased sharply as well: each month we can examine over 6 billion views of text, image, and video content created by BuzzFeed in addition to hundreds of billions of data points from third party sources like Facebook Instant Articles and Snapchat.
We’ve found ourselves tackling hard to solve problems, finding surprising (and sometimes entertaining) answers, some of which I’d like to share with you here. Below is the framework on which we’ve built our data science organization, and some insights we’ve learned that I hope will be helpful as we move more fully into the world of social platforms and apps.
Anonymize all usage data
First and foremost, we respect the privacy of data. At BuzzFeed, our policy is simple: we anonymize all usage data, have strict internal policies around our employees only accessing data in aggregate form, and are building technical safeguards that would alert us if that policy is breached. We do this because we don’t care about individuals, we care about what we can see from groups of people that point to a trend or pattern we can learn from.
Embrace complexity
Recent debates about the most important or newest web metrics do not distract us. Unique visitors matter, shares matter, front page visits matter, app DAUs (Daily Active Users) and MAUs (Monthly Active Users) matter, social media followers matter, diversity of traffic sources matters, time spent matters, editorial judgement matters, UX, design and brand perception matter, press pick-up and moving-the-conversation matters, scoops matter, diversity of content matters, and we are probably missing a few others. In other words — it’s a long list.
BuzzFeed is a combination of art, science, and good judgement. Understanding that balance is a competitive advantage.
To measure the overall reach of the company, we look at a combination of metrics that are available across platforms. Here is a sampling of what that data looks like.
Content views are views of BuzzFeed content (videos, articles, lists, illustrations) regardless of the platform on which the content lives. Not included are homepage or feed views, or impressions of link promotions on social networks.
As we all know, Facebook, YouTube, and Snapchat all count video views in different ways. Hopefully though, they count minutes in the same way, so time spent helps us understand more about what our audience is doing.
We can look at referral sources and platform locations to see which ones over-index and which ones under-index for time spent.
November–December 2015 data
We define subscribers as people who, by taking an action, have shown an interest in the BuzzFeed brand — such as people who use our mobile apps, sign up for our newsletters, visit our homepage, or follow our social feeds. This helps us understand different relationships with our audiences and how they are growing.
Lastly, we are starting to look at engagements, defined as shares, hearts, comments, likes, repins, etc., on all the platforms. In the past we avoided using this metric, because we felt that the share data provided by Facebook about articles was too unreliable. But the number of Facebook shares of articles, while still very large, represents a smaller and smaller portion of our total engagements, so now we will start examining this.
Be humble: Data is best used for learning, not vanity
Now that I’ve shown some really big numbers about BuzzFeed’s reach, I’ll recommend that we don’t get too high on ourselves. Knowing our top-line numbers is useful for understanding large trends and for bragging (yes, we do brag!), but it doesn’t help us make better content or connect with our audiences. Ultimately, the reason we care about data is that we hope to learn something from it. We should look to other, smaller numbers for that.
Questions matter more than answers
If you don’t ask the right questions, then you won’t get useful answers. Yet there is an all-too-common assumption that the “standard” questions are the only ones that can be asked. Suppose an editor, wanting to know how his stories are doing, asks for a page-view report. A “web analytics” team could simply pull this data and send it to him. At BuzzFeed, the data science team handles web analytics; so even before pulling the data, we will discuss with the editor what, intuitively, she is trying to understand, and then figure out which metrics best measure that. And if we’re not currently gathering data for those metrics, we can take steps to start doing so. This is the simplest example. As problems get more complex, asking the right questions matters even more.
Be skeptical about the data
There is a sadly pervasive belief that data = truth. If numbers are involved, it must be true, and the more numerous the numbers, the truer the truth! The cult of “big data” assumes that with volume of data comes trustworthiness. The reality is that every data collection scheme is a set of rules coded by humans; any experiment could hide inherent biases; every model’s assumptions could be wrong. If the methodology is faulty, then it doesn’t matter how much data you have. Size doesn’t trump technique; both matter. Data scientists are duty-bound to question the viability of data sets, to reconsider methods of analysis, and to question the degree of “truth” that can be extracted. Only then can we get closer to understanding what is happening, which is always more complex than a single number can show.
Data can tell you what happened, but rarely why
Let’s say we’ve asked the pertinent questions, set up the least biased experiments, and analyzed the optimal way. Fabulous — we know something! While we have figured out something that happened, we shouldn’t assume that we know why. We can certainly speculate, and design further experiments to test hypotheses, and even ask users with surveys, but it’s always unproductive (and usually counter-productive) to think we know more than we actually do.
Sometimes a lot of data will tell you what will likely happen. Predictive analysis is one of our core areas of research. Again, we can create a predictive algorithm that works well and is based on correlations seen in the data, but it doesn’t mean we understand the “why” of what we’re trying to predict. Correlation and causation are not the same; and we need to think about when it makes sense to act on correlation
Data is only as powerful as the organization behind it
BuzzFeed has a thriving, effective data science team because the culture of the company allows it. Some examples of how culture is critical to the success of data science:
- The whole company is aligned along a clearly articulated strategy, so the questions that are asked have some of this long-term thinking in mind.
- Both editorial and business teams are lean and experimental, so they can test data hypotheses fairly quickly. Flexibility is key: having the data is pointless if you can’t use the data; as is speed: having the data is equally pointless if you can’t use it before it becomes outdated.
- We’ve invested in a technology infrastructure that can support data science needs: frameworks for large-scale collection and processing of data, tools and APIs (Application Program Interface) for obtaining and analyzing data, ad-hoc data stores for analyses, and an A/B testing platform.
- Employees in every group and at every level are aware that data (and, more broadly, technology) are core to our success. In fact, all employees get trained on BuzzFeed’s approach to data and our home grown technology at orientation. They also know that data has limits, which leads to the next point.
Be pragmatic: Look at the metric(s) that helps you learn about your platform or achieve your objectives.
Knowing what you’re trying to do or learn is the first step in figuring out what metrics to look at. (In business speak, I would say: Identify your Key Performance Indicators (KPIs) in advance.) We don’t use the same metrics for success on all platforms. We don’t use the same metrics for success for all kinds of videos. We don’t use the same metrics for success for all kinds of articles, or for all of our Facebook pages. It would be easier if we did! But we are trying to learn and achieve different things with each platform, page type and video/post type. The same is true with our advertisers. Each advertiser has its particular goals (e.g., maybe one is more interested in scale while another is more interested in Direct Response) and metrics, and what you’re optimizing for should reflect that.
This sounds like it’s complicated and messy, and it is! But it’s also pragmatic. For example, for certain kinds of videos, we look at views on YouTube but shares on Facebook. We found that different metrics gave clearer signals on these different platforms.
Data should inform your choices, not determine your strategy. In fact, over-optimization can lead to achieving only a local maximum.
Be human: Some kinds of impact are not quantifiable
- A Texas woman who was sentenced to 45 years in prison for failing to protect her son from her abusive boyfriend, even though she tried to stop the beating, was granted parole after being featured in a 2014 BuzzFeed News investigation.
- The U.N. Human Rights office and the NYC Human Resources Administration (the largest social services agency in the country) included our video “What It’s Like to Be Intersex” in its LGBTQI (Lesbian, Gay, Bi, Trans, Queer and Intersex) trainings.
- Our Snapchat edition dedicated to Muslim identity touched a lot of people. We received lots of tweets and this email: “I’m a Muslim girl living in Australia facing the hardship of trying to explain my religion to my friends and colleagues almost every day and I always get called brainwashed for following my religion. Clicking on BuzzFeed today and seeing a whole series dedicated to Muslims… Words can’t start to explain how much that meant to me.”
We can measure some kinds of impact, and we do it regularly for our advertisers, especially since they have clear objectives. But some kinds of impact are not quantifiable in the sense that you can’t say that one instance is larger than another. And trying to do so diminishes our ability to make a difference and connect with people-
Data is under-utilized and over-hyped
Today, it’s hard to find a media organization that isn’t thinking about data science at some level. People talk about big data, small data, lean data, smart data. We try to not get caught up in the labeling. We try to focus on the problems we’re solving. The only way for media organizations to get the most out of data science is to keep questioning, collecting, scrubbing, learning, analyzing, testing, making mistakes, and doing it again.
In conclusion
Metrics should reflect what a company cares about, and each media company has to choose its own data points that matter. Even now, as BuzzFeed adopts a “global cross-platform” strategy where we continuously test, learn and publish across multiple languages, editions and platforms, we are dreaming up new ways to understand and learn from data. What if we could calculate a cross-platform lift for each piece of content or each content frame? What if we could predict the ROI (Return on Investment) of translating a piece of content into a particular language? What if we could do the same for advertisers? It’s an exciting time!